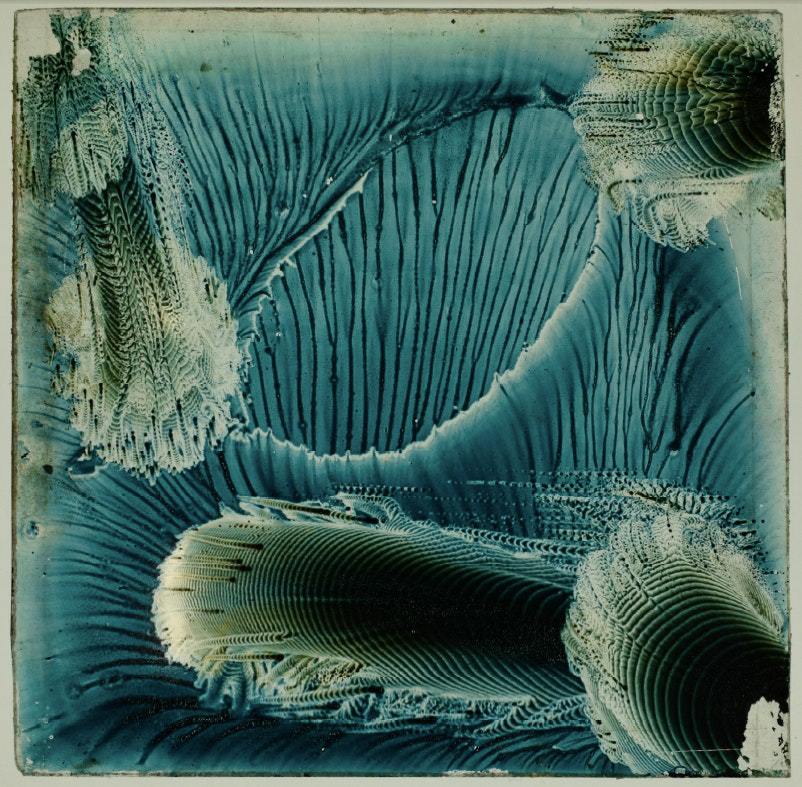
As more countries realize the potential AI has to offer in terms of economic opportunities, large societal problems have also been lumped under the category of things that can be “solved” using AI. This is reflected in the national AI strategies of various countries1 where grandiose claims are made that if only we throw enough computation, data, and the pixie dust of AI on it, we will be able to solve, among other things, the climate crises that looms large over our heads.
AI systems are not without their flaws. There are many ethical issues to consider when thinking about deploying AI systems into society—particularly environmental impacts3.
Of course, readers of this publication are no strangers to such grandiose claims every time there is a new policy or technical instrument2 that is proposed and ends up falling short of meaningfully addressing the climate crisis. There is no silver bullet!
The Case for Considering the Carbon Footprint of AI
With any process, technology, or tool, we should always think about externalities that it levies and contrast that with the benefits that it offers.
AI is not different in that regard. There are many reasons to pay attention to the carbon impact of AI systems, especially as larger and larger models are becoming the norm to achieve state-of-the-art results in the industry. One only needs to take a look at the immense coverage that the release of Generative Pre-trained Transformer 3 (GPT-3)4 got in terms of what it was capable of achieving5. It wasn’t just impressive that it beat a lot of benchmarks but also the variety of tasks that it was able to accomplish including powering up virtual characters in games to have more natural conversations with players that aren’t scripted among many other impressive feats6. It clocks in at 175 billion parameters and is certainly quite large. But, the trend towards ever-larger models continues with Google’s release of the Switch Transformer that clocked in at 1.6 trillion parameters7.
Estimates place the cost of training GPT-3 at several million dollars8. Therein lies the first issue with AI systems: they become incredibly expensive to train. It thus limits access to a select few who work at large research labs in academia or industry that have access to the financial resources that fund the computing and data infrastructure needed to bootstrap and develop these systems. But, we’ll come back to this point and other social considerations in later sections.
Then there is the massive environmental impact that training such large models have: hundreds of thousands of pounds of CO2eq are emitted, comparable to the lifetime carbon emissions of several cars9.
We should adopt a lifecycle approach to AI systems when evaluating their environmental impact.
While not all of us are going to be building such large models, there is enough work done at a large-scale that it behooves us to consider what is the cost-benefit tradeoff when we continue to harness large-scale models as a way forward in achieving state-of-the-art results.
More so, it is not just the environmental impact at the time of training but also at inference time (even though it is a tiny fraction of the training cost, run a large number of times, it can become sizable) that should be taken into account. Essentially, we should adopt a lifecycle approach to AI systems when evaluating their environmental impact. This has just been the case with other products as well where we seek to assess what impact they will have on the environment when we want to assess how eco-friendly they are10.
The Current State of Carbon Accounting for AI Systems
Initiatives like Green AI11 advocate for including efficiency alongside accuracy and other evaluation metrics when building AI systems. Other initiatives propose strategies like real-time energy-use tracking that can help mitigate carbon emissions and reduction of energy consumption12. Experiment impact trackers13 realize this approach through the use of drop-in functions that can process log files to generate carbon impact statements. There are online tools like MLCO2 calculator14 that require developers to input parameters that capture the specific hardware that they used, the cloud provider, the datacenter region, and the number of hours trained to provide an estimate for the carbon emissions.
However, they suffer from 2 core problems: they compel developers to exit their natural workflow and enter information that they have to hunt from web portals and track manually, and they rely on statically sourced energy generation mix data which doesn’t always give the most accurate picture. In fact, post-hoc tracking can introduce measurement errors that are off by more than an order of a magnitude as discovered in this recently published paper15, which can have significant implications for any work that seeks to provide useful insights to practitioners.
With regards to the first problem, code-based tools like CodeCarbon16 and CarbonTracker17 alleviate the problem of the natural workflow disruption, with CodeCarbon now offering integration with CometML18 that further naturalizes the inclusion of such metrics with the rest of the data science and machine learning workflows and artefacts gathering. The second problem is a little bit harder to solve and continues to remain a challenge which the larger climate tech community is working towards solving. Having access to energy mix in real-time, especially with the rising use of renewables and special agreements that data centers have with power grids will provide more fine-grained information to data scientists and machine learning practitioners which could impact model architecture choices in addition to when they choose to run their training jobs.
There is also a lot of debate in terms of what are the most accurate and representative ways of measuring the carbon impact of AI, with some advocating for CO2eq that are calculated from power consumption while others focusing on floating point operations. This bears similarity to problems faced in the broader field of carbon impact measurement19 and hence we might be able to take lessons from that rich body of literature and applied practice to inform our approach in the field of AI. There is a lot to be learned from adjacent fields and this is our opportunity to do so.
Environmental Impact Concerns Need to be Integrated with Other Social Impacts of AI Discussions
Access to large compute and data infrastructure comes with a strong correlation with demographic homogeneity that skews away disproportionately from minoritzed groups20. In addition, such approaches also require large amounts of shadow labor and ghost work21 to empower them. The brunt of which again falls on the shoulders of the marginalized peoples of the world. Some of this work can have tremendous negative consequences in terms of mental health as the workers might be forced, as a part of their job, to label content that can have damaging psychological consequences22.
More so, the collection of data that is required to power these models in the first place takes its roots in exploitation of end-users whose privacy is violated and whose consent is ignored or elicited under pretenses of providing meaningful services23. Research has shown that users aren’t always capable of providing informed consent when their data is being used24.
Given the large body of research in the field of the societal impact of AI, coupled with grassroots initiatives and imminent legislation considering the environmental impact concerns can help bring momentum to the environmental considerations and make them a normal part of the discourse rather than an esoteric field of pursuit (as is still the case at the time of writing this). It also has the benefit of piggybacking on some of the emerging instruments to jumpstart more actionable and practical implications rather than having to reinvent the wheel from scratch.
Our goal with this is to create more empowered developers and users of AI systems so that they can utilize, among other mechanisms, social pressure through their demands for more green AI systems.
Our goal with this is to create more empowered developers and users of AI systems so that they can utilize, among other mechanisms, social pressure through their demands for more green AI systems.
Such approaches have shown to be effective in creating change when it comes to shifting supply chain patterns and development behaviour in other fields25.
The SECure Framework
The SECure framework is built keeping in mind these ideas with its primary focus being on providing actionable insights to practitioners and consumers of AI systems to trigger behaviour change which is the major missing element in the current tooling that seeks to measure carbon impacts of AI systems. It ties together the social and environmental considerations with a view that they are mutually reinforcing and will lead to faster and more sustainable adoption of these ideas in practice.
It consists of 4 pillars that we will explore in some detail in the following sections. The background to keep in mind for them is that at every step of the way, we are focused on two key aspects: minimizing friction to existing workflows to boost adoption of these processes and providing actionable insights that can trigger behaviour change to achieve the goal of building more eco-socially responsible AI systems.
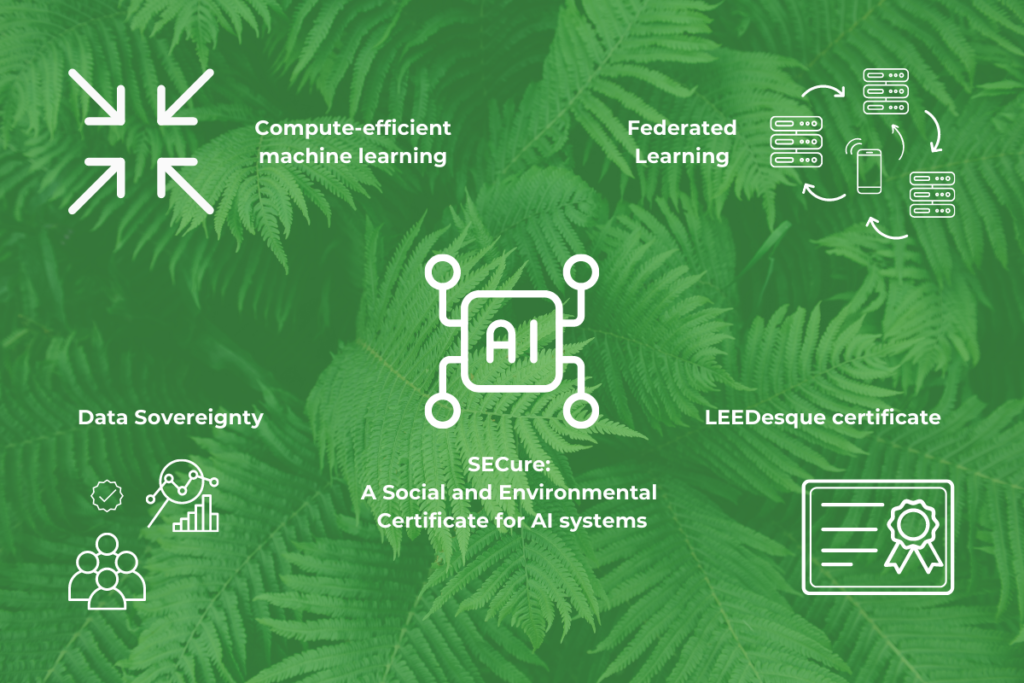
The approach adopted in the framework pays heed to the larger social concerns of environmentally-insensitive AI systems with the aim of leveraging the momentum in that domain for increased adoption and adding more justification (in places that seek it) to pay attention to these concerns as legitimate threats to building ethical, safe, and inclusive AI systems.
Compute-Efficient Machine Learning
Using compute-efficient machine learning methods has the potential to lower the computation burdens that typically make access inequitable for researchers and practitioners who are not associated with large organizations that have access to heavy compute and data processing infrastructures. As an example, recent advances in the quantization of computations in neural networks26 have led to significant decreases in computational requirements. This also allows for the use of lower-cost resources like CPUs compared to more expensive hardware for the training of complex architectures which typically require GPUs or TPUs. Altering data layouts, computation batching, and fixed-point instructions can also speed up computations on CPUs making AI more accessible to those who are restricted in their access to computing resources27.
Another area of ML research that has bearing for compute-efficient machine learning is that of machine learning models for resource-constrained devices like edge-computing on Internet of Things (IoT) devices. For example, with devices that have RAM sizes in KB, model size can be minimized along with prediction costs using approaches like Bonsai28 that proposes a shallow, sparse tree-based algorithm. Another approach is called ProtoNN that is inspired by kNN but uses minimal computation and memory to make real-time predictions on resource-constrained devices29. Novel domain-specific languages like SeeDot30, which expresses ML-inference algorithms and then compiles that into fixed points, makes these systems amenable to run on edge-computing devices. Other distilled versions of large-scale networks like MobileNets31 and the growing prevalence of TinyML32 will also bring about cost- and compute-efficiency gains. This part of the framework proposes the computation of a standardized metric that is parametrized by the above components as a way of making quantified comparisons across different hardware and software configurations allowing people to make informed decisions in picking one solution over another.
Federated Learning
As a part of this framework, the utilization of federated learning33 approaches as a mechanism to do on-device training and inference of ML models will also be beneficial. The purpose of utilizing this technique is to mitigate risks and harm that arises from centralization of data, including data breaches and privacy intrusions.
These are known to fundamentally harm the trust levels that people have in technology and are typically socially-extractive given that they may use data for more than the purposes specified when the data is sourced into a single, centralized source. Federated learning also has the second-order benefit of enabling computations to run locally thus potentially decreasing carbon impacts if the computations are done in a place where electricity is generated using clean sources34. It may be that there are gains to be had from an “economies of scale” perspective when it comes to energy consumption in a central place—like for a data center that relies on government-provided access to clean energy. This is something that still needs to be validated, but the benefits in terms of reducing social harm are definite, and such mechanisms provide for secure and private methods for working on data that constitutes personally identifiable information (PII).
Data Sovereignty
Data sovereignty refers to the idea of strong data ownership and giving individuals control over how their data is used, for what purposes, and for how long. It also allows users to withdraw consent for use if they see fit. In the domain of machine learning, especially when large datasets are pooled from numerous users, the withdrawal of consent presents a major challenge.
Specifically, there are no clear mechanisms today that allow for the removal of data traces or of the impacts of data related to a user in a meaningful manner from a machine learning system without requiring a retraining of the system. Preliminary work35 in this domain showcases some techniques for doing so—yet, there is a lot more work needed in this domain before this can be applied across the board for the various models that are used. Thus, having data sovereignty at the heart of system design which necessitates the use of techniques like federated learning is a great way to combat socially-extractive practices in machine learning today.
Data sovereignty also has the second-order effect of respecting differing norms around data ownership which are typically ignored in discussions around diversity and inclusion as it relates to the development of AI systems. For example, indigenous perspectives on data36 are quite different and ask for data to be maintained on indigenous land, used and processed in ways that are consistent with their values.
LEEDesque certificate
The certification model today relies on some sort of a trusted, third-party, independent authority that has the requisite technical expertise to certify the system meets the needs as set out in standards, and that is if there are any that are widely accepted. Certificates typically consist of having a reviewer who assesses the system to see if it meets the needs as set out by the certifying agency. The organization is then issued a certificate if they meet all the requirements.
An important, but seldom discussed component of certification is something called the Statement of Applicability (SoA). Certificates are limited in terms of what they assess. What the certifying agency chooses to evaluate, and the inherent limitation that these choices are representative of the system at a particular moment in time with a particular configuration. What gets left out of the conversation is the SoA and how much of the system was covered under the scope of evaluation. Often the SoA is also not publicly or easily available, while the certification mark is shared widely to signal to consumers that the system meets the requirements as set out by the certification authority. Without the SoA, one cannot really be sure of what parts of the system were covered. This might be quite limiting in a system that uses AI, as there are many points of integration as well as pervasive use of data and inferences made from the data in various downstream tasks.
Best Practices to Make Certificates More Effective
Recognizing some of the pitfalls in the current mechanisms for certification, the certification body should bake in the SoA into the certificate itself such that there is not a part of the certification that is opaque to the public.
Secondly, given the fast-evolving nature of the system, especially in an online-learning environment for machine learning applications, the certificate has a very short lifespan. An organization would have to be recertified so that the certificate reflects as accurately as possible the state of the system in its current form. Certification tends to be an expensive operation and can thus create barriers to competitiveness in the market where only large organizations are able to afford the expenses of having their systems certified.
To that end, having the certification process be automated as much as possible to reduce administrative costs—as an example, having mechanisms like Deon37 might help. Also, tools that would enable an organization to become compliant for a certification should be developed and made available in an open-source manner to further encourage organizations to take this on and make it a part of their regular operations.
Standardized Measurement Technique
Standardization will also serve to allow for multiple certification authorities to offer their services, thus further lowering the cost barriers and improving market competitiveness while still maintaining an ability to compare across certificates provided by different organizations. An additional measure that will be of utmost importance is to have the certificate itself be intelligible to a wide group of people. It should not be arcane and prevent people from understanding the true meaning and impact of the certification. It will also empower users to make choices that are well-informed.
Survey Component
To build on the point made above, the goal of the certification process is to empower users to be able to make well-informed choices. Identifying what information users are seeking from certification marks and how that information can be communicated in the most effective manner is critical for the efficacy of the certificate. Additionally, triggering behaviour change on the part of the users through better-informed decisions on which products/services to use needs to be supplemented with behaviour change on the part of the organizations building these systems. Clear comparison metrics that allow organizations to assess the state of their systems in comparison with actors in the ecosystem will also be important. Keeping that in mind, a survey of the needs of practitioners will help ensure the certification is built in a manner that meets their needs head-on, thereby encouraging widespread adoption.
Here’s what we can do next
Hopefully, this whirlwind tour through the idea of SECure38 has excited you to take some action! There is a lot of work yet to be done, a lot of refinement that is needed before we get to a place where we can build such eco-socially responsible AI systems as a normalized practice in a frictionless fashion.
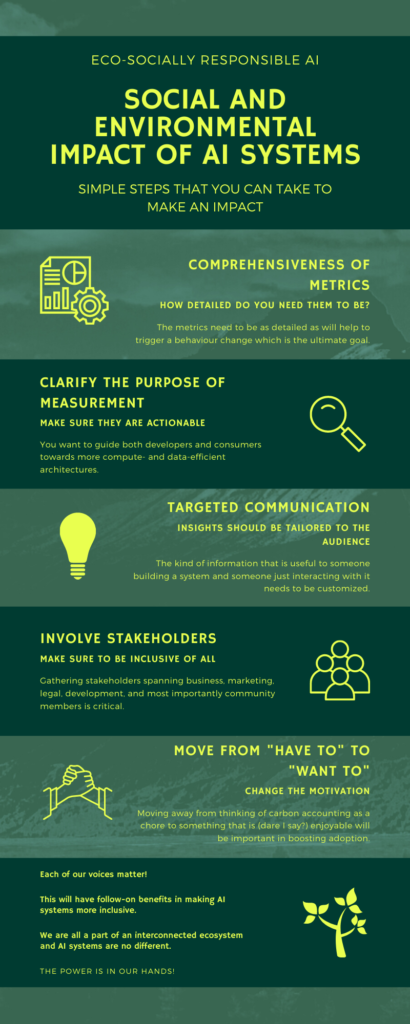
Some actions that I believe will make an impact include:
- Examining what is the degree of comprehensiveness and granularity needed on the carbon measurement metrics. In essence, our goal is to shift behaviour and not measure just for measurement’s sake. If we are able to shift behaviour with coarse estimates of carbon emissions, perhaps we can do away with some of the problems around being able to source real-time data for energy mix and the different computational approaches to assessing the energy use.
- Clarifying further that the purpose of measurement is to alter behaviour, both of the developers in choosing more compute- and data-efficient architectures and systems, and for the consumers in picking offerings that are more eco-friendly.
- The results, whether presented through a certificate, a badge, or by some other means, need to present information in a way that is tailored to its target audience. Information shared with a developer won’t have the same impact on a consumer who is perhaps less savvy about the internal workings of the system and requires a different level of abstraction to make a decision.
- Involving the right stakeholders is also critical in garnering momentum for such an endeavor. This includes not only folks from the development side, including data scientists and machine learning engineers, but also designers, marketers, business executives, and most importantly community stakeholders who will be the ultimate recipients of the outputs of the system. They can help inform what will form effective approaches to meet the goals outlined in the previous points.
- Finally, and perhaps most importantly, we need to find ways that help to convert this from something that is a “chore” that needs to be performed to something that stakeholders want to perform. This is critical to galvanize action – climate change has faced inertia in people acting, amongst many reasons, due to the tasks required as a part of being more eco-friendly to be too onerous39.
A word of caution of course remains that none of this is a replacement for first-principles thinking on whether a system needs to be built in the first place, whether it respects the rights, aspirations, culture, and context of the community that it is meant to serve. Finally this approach is not a replacement for consulting domain experts and working with community stakeholders on the ground who have lived experiences and are the closest to those that you are looking to serve through your AI system.
In support of the points made so far, investing in public facilities for computing and data infrastructure such as data commons40 and national public clouds can enable this progress, democratizing the field further. To further related equity goals and address power imbalances, the notion of data co-operatives41 can empower people to build local solutions to their problems. Finally, a shift in the research and development in the field towards small-data and meta-learning approaches can also further these goals.
Given the strong influence that market forces have on which solutions are developed and deployed, the SECure certificate can serve as a mechanism creating the impetus for consumers and investors to demand more transparency on the social and environmental impacts of these technologies and then use their purchasing power to steer the progress of development in this field that accounts for these impacts. Responsible AI investment, akin to impact investing, will be easier with a mechanism that allows for standardized comparisons across various solutions, which is what SECure is perfectly geared towards.
You can stay in touch about this work by signing up at https://greenml.substack.com
About the author
Abhishek Gupta is the Founder and Principal Researcher at the Montreal AI Ethics Institute and a Machine Learning Engineer at Microsoft where he also serves on the Responsible AI Board for Commercial Software Engineering (CSE). His work focuses on applied technical and policy measures to build ethical, safe, and inclusive AI systems. You can find out more about his work at Abhishek Gupta | AI Ethics Researcher | Machine Learning Engineer.
References
[1] https://oecd.ai
[2] Arvesen, A., Bright, R. M., & Hertwich, E. G. (2011). Considering only first-order effects? How simplifications lead to unrealistic technology optimism in climate change mitigation. Energy Policy, 39(11), 7448-7454.
[3] Ouchchy, L., Coin, A., & Dubljević, V. (2020). AI in the headlines: the portrayal of the ethical issues of artificial intelligence in the media. AI & SOCIETY, 35(4), 927-936.
[4] Brown, T. B., Mann, B., Ryder, N., Subbiah, M., Kaplan, J., Dhariwal, P., … & Amodei, D. (2020). Language models are few-shot learners. arXiv preprint arXiv:2005.14165.
[6] https://openai.com/blog/gpt-3-apps/
[7] Fedus, W., Zoph, B., & Shazeer, N. (2021). Switch Transformers: Scaling to Trillion Parameter Models with Simple and Efficient Sparsity. arXiv preprint arXiv:2101.03961.
[8] https://venturebeat.com/2020/06/01/ai-machine-learning-openai-gpt-3-size-isnt-everything/
[9] Strubell, E., Ganesh, A., & McCallum, A. (2019). Energy and policy considerations for deep learning in NLP. arXiv preprint arXiv:1906.02243.
[10] Giudice, F., La Rosa, G., & Risitano, A. (2006). Product design for the environment: a life cycle approach. CRC press.
[11] Schwartz, R., Dodge, J., Smith, N. A., & Etzioni, O. (2019). Green AI; 2019. arXiv preprint arXiv:1907.10597.
[12] Henderson, P., Hu, J., Romoff, J., Brunskill, E., Jurafsky, D., & Pineau, J. (2020). Towards the systematic reporting of the energy and carbon footprints of machine learning. Journal of Machine Learning Research, 21(248), 1-43.
[13] https://github.com/Breakend/experiment-impact-tracker
[14] https://mlco2.github.io/impact/
[15] Patterson, D., Gonzalez, J., Le, Q., Liang, C., Munguia, L. M., Rothchild, D., … & Dean, J. (2021). Carbon Emissions and Large Neural Network Training. arXiv preprint arXiv:2104.10350.
[16] https://github.com/mlco2/codecarbon
[17] https://github.com/lfwa/carbontracker
[18] https://www.comet.ml/site/
[19] Laurent, A., Olsen, S. I., & Hauschild, M. Z. (2012). Limitations of carbon footprint as indicator of environmental sustainability. Environmental science & technology, 46(7), 4100-4108.
[20] https://en.wiktionary.org/wiki/minoritize
[21] Gray, M. L., & Suri, S. (2019). Ghost work: How to stop Silicon Valley from building a new global underclass. Eamon Dolan Books.
[22] Roberts, S. T. (2014). Behind the screen: The hidden digital labor of commercial content moderation (Doctoral dissertation, University of Illinois at Urbana-Champaign).
[23] Bouguettaya, A. R. A., & Eltoweissy, M. Y. (2003). Privacy on the Web: facts, challenges, and solutions. IEEE Security & Privacy, 1(6), 40-49.
[24] Bashir, M., Hayes, C., Lambert, A. D., & Kesan, J. P. (2015). Online privacy and informed consent: The dilemma of information asymmetry. Proceedings of the Association for Information Science and Technology, 52(1), 1-10.
[25] Hainmueller, J., Hiscox, M. J., & Sequeira, S. (2015). Consumer demand for fair trade: Evidence from a multistore field experiment. Review of Economics and Statistics, 97(2), 242-256.
[26] Jacob, B., Kligys, S., Chen, B., Zhu, M., Tang, M., Howard, A., … & Kalenichenko, D. (2018). Quantization and training of neural networks for efficient integer-arithmetic-only inference. In Proceedings of the IEEE Conference on Computer Vision and Pattern Recognition (pp. 2704-2713).
[27] Vanhoucke, V., Senior, A., & Mao, M. Z. (2011). Improving the speed of neural networks on CPUs.
[28] Kumar, A., Goyal, S., & Varma, M. (2017, July). Resource-efficient machine learning in 2 kb ram for the internet of things. In International Conference on Machine Learning (pp. 1935-1944). PMLR.
[29] Gupta, C., Suggala, A. S., Goyal, A., Simhadri, H. V., Paranjape, B., Kumar, A., … & Jain, P. (2017, July). Protonn: Compressed and accurate knn for resource-scarce devices. In International Conference on Machine Learning (pp. 1331-1340). PMLR.
[30] Gopinath, S., Ghanathe, N., Seshadri, V., & Sharma, R. (2019, June). Compiling kb-sized machine learning models to tiny iot devices. In Proceedings of the 40th ACM SIGPLAN Conference on Programming Language Design and Implementation (pp. 79-95).
[31] Howard, A. G., Zhu, M., Chen, B., Kalenichenko, D., Wang, W., Weyand, T., … & Adam, H. (2017). Mobilenets: Efficient convolutional neural networks for mobile vision applications. arXiv preprint arXiv:1704.04861.
[33] Bonawitz, K., Eichner, H., Grieskamp, W., Huba, D., Ingerman, A., Ivanov, V., … & Roselander, J. (2019). Towards federated learning at scale: System design. arXiv preprint arXiv:1902.01046.
[34] Qiu, X., Parcollet, T., Beutel, D., Topal, T., Mathur, A., & Lane, N. (2020, December). Can Federated Learning Save the Planet?. In NeurIPS-Tackling Climate Change with Machine Learning.
[35] Bourtoule, L., Chandrasekaran, V., Choquette-Choo, C. A., Jia, H., Travers, A., Zhang, B., … & Papernot, N. (2019). Machine unlearning. arXiv preprint arXiv:1912.03817.
[36] Kukutai, T., & Taylor, J. (2016). Indigenous data sovereignty: Toward an agenda. Anu Press.
[37] https://deon.drivendata.org/
[38] Gupta, A., Lanteigne, C., & Kingsley, S. (2020). SECure: A Social and Environmental Certificate for AI Systems. arXiv preprint arXiv:2006.06217.
[39] Wagner, G., & Zeckhauser, R. J. (2012). Climate policy: hard problem, soft thinking. Climatic change, 110(3), 507-521.
[40] Miller, P., Styles, R., & Heath, T. (2008). Open Data Commons, a License for Open Data. LDOW, 369.
[41] Hafen, E., Kossmann, D., & Brand, A. (2014). Health data cooperatives-citizen empowerment. Methods Inf Med, 53(2), 82-86.